What is the fastest SDC variant?¶
In this project, we test different variants of SDC for a particular problem to see which one is the fastest. More precisely, we run SDC for the 1D Fisher equation and the 2D Gray-Scott problem with PETSc data types and solvers, using fully implicit, semi-implicit and multi-implicit time-stepping. We also test exact spatial solves vs. inexact ones (aka inexact SDC, ISDC)
Fisher and Gray-Scott equations¶
The two run scripts simply test all variants of SDC after the other, each of them exact and then inexact. The results are gathered, stored and shown in comparison. Note that on standard machines the inexact semi-implicit variant wins each time (may be different for the CI testing).
Full code: pySDC/projects/SDC_showdown/SDC_timing_Fisher.py
import os
import pickle
import numpy as np
import pySDC.helpers.plot_helper as plt_helper
from pySDC.helpers.stats_helper import get_sorted
from pySDC.implementations.controller_classes.controller_nonMPI import controller_nonMPI
from pySDC.implementations.problem_classes.GeneralizedFisher_1D_PETSc import (
petsc_fisher_multiimplicit,
petsc_fisher_fullyimplicit,
petsc_fisher_semiimplicit,
)
from pySDC.implementations.sweeper_classes.generic_implicit import generic_implicit
from pySDC.implementations.sweeper_classes.imex_1st_order import imex_1st_order
from pySDC.implementations.sweeper_classes.multi_implicit import multi_implicit
def setup_parameters():
"""
Helper routine to fill in all relevant parameters
Note that this file will be used for all versions of SDC, containing more than necessary for each individual run
Returns:
description (dict)
controller_params (dict)
"""
# initialize level parameters
level_params = dict()
level_params['restol'] = 1e-06
level_params['dt'] = 0.25
level_params['nsweeps'] = [1]
# initialize sweeper parameters
sweeper_params = dict()
sweeper_params['quad_type'] = 'RADAU-RIGHT'
sweeper_params['num_nodes'] = [3]
sweeper_params['Q1'] = ['LU']
sweeper_params['Q2'] = ['LU']
sweeper_params['QI'] = ['LU']
sweeper_params['initial_guess'] = 'zero'
# initialize problem parameters
problem_params = dict()
problem_params['nu'] = 1
problem_params['nvars'] = 2049
problem_params['lambda0'] = 2.0
problem_params['interval'] = (-50, 50)
problem_params['nlsol_tol'] = 1e-10
problem_params['nlsol_maxiter'] = 100
problem_params['lsol_tol'] = 1e-10
problem_params['lsol_maxiter'] = 100
# initialize step parameters
step_params = dict()
step_params['maxiter'] = 50
# initialize space transfer parameters
# space_transfer_params = dict()
# space_transfer_params['finter'] = True
# initialize controller parameters
controller_params = dict()
controller_params['logger_level'] = 30
# fill description dictionary for easy step instantiation
description = dict()
description['problem_class'] = None # pass problem class
description['problem_params'] = problem_params # pass problem parameters
description['sweeper_class'] = None # pass sweeper (see part B)
description['sweeper_params'] = sweeper_params # pass sweeper parameters
description['level_params'] = level_params # pass level parameters
description['step_params'] = step_params # pass step parameters
# description['space_transfer_class'] = mesh_to_mesh_petsc_dmda # pass spatial transfer class
# description['space_transfer_params'] = space_transfer_params # pass paramters for spatial transfer
return description, controller_params
def run_SDC_variant(variant=None, inexact=False):
"""
Routine to run particular SDC variant
Args:
variant (str): string describing the variant
inexact (bool): flag to use inexact nonlinear solve (or nor)
Returns:
timing (float)
niter (float)
"""
# load (incomplete) default parameters
description, controller_params = setup_parameters()
# add stuff based on variant
if variant == 'fully-implicit':
description['problem_class'] = petsc_fisher_fullyimplicit
description['sweeper_class'] = generic_implicit
elif variant == 'semi-implicit':
description['problem_class'] = petsc_fisher_semiimplicit
description['sweeper_class'] = imex_1st_order
elif variant == 'multi-implicit':
description['problem_class'] = petsc_fisher_multiimplicit
description['sweeper_class'] = multi_implicit
else:
raise NotImplementedError('Wrong variant specified, got %s' % variant)
if inexact:
description['problem_params']['nlsol_maxiter'] = 1
out = 'Working on inexact %s variant...' % variant
else:
out = 'Working on exact %s variant...' % variant
print(out)
# set time parameters
t0 = 0.0
Tend = 1.0
# instantiate controller
controller = controller_nonMPI(num_procs=1, controller_params=controller_params, description=description)
# get initial values on finest level
P = controller.MS[0].levels[0].prob
uinit = P.u_exact(t0)
# call main function to get things done...
uend, stats = controller.run(u0=uinit, t0=t0, Tend=Tend)
# compute exact solution and compare
uex = P.u_exact(Tend)
err = abs(uex - uend)
# filter statistics by variant (number of iterations)
iter_counts = get_sorted(stats, type='niter', sortby='time')
# compute and print statistics
niters = np.array([item[1] for item in iter_counts])
out = ' Mean number of iterations: %4.2f' % np.mean(niters)
print(out)
out = ' Range of values for number of iterations: %2i ' % np.ptp(niters)
print(out)
out = ' Position of max/min number of iterations: %2i -- %2i' % (int(np.argmax(niters)), int(np.argmin(niters)))
print(out)
out = ' Std and var for number of iterations: %4.2f -- %4.2f' % (float(np.std(niters)), float(np.var(niters)))
print(out)
print('Iteration count (nonlinear/linear): %i / %i' % (P.snes_itercount, P.ksp_itercount))
print(
'Mean Iteration count per call: %4.2f / %4.2f'
% (P.snes_itercount / max(P.snes_ncalls, 1), P.ksp_itercount / max(P.ksp_ncalls, 1))
)
timing = get_sorted(stats, type='timing_run', sortby='time')
print('Time to solution: %6.4f sec.' % timing[0][1])
print('Error vs. PDE solution: %6.4e' % err)
print()
assert err < 9.2e-05, 'ERROR: variant %s did not match error tolerance, got %s' % (variant, err)
assert np.mean(niters) <= 10, 'ERROR: number of iterations is too high, got %s' % np.mean(niters)
return timing[0][1], np.mean(niters)
def show_results(fname):
"""
Plotting routine
Args:
fname: file name to read in and name plots
"""
file = open(fname + '.pkl', 'rb')
results = pickle.load(file)
file.close()
plt_helper.mpl.style.use('classic')
plt_helper.setup_mpl()
plt_helper.newfig(textwidth=238.96, scale=1.0)
xcoords = list(range(len(results)))
sorted_data = sorted([(key, results[key][0]) for key in results], reverse=True, key=lambda tup: tup[1])
heights = [item[1] for item in sorted_data]
keys = [(item[0][1] + ' ' + item[0][0]).replace('-', '\n') for item in sorted_data]
plt_helper.plt.bar(xcoords, heights, align='center')
plt_helper.plt.xticks(xcoords, keys, rotation=90)
plt_helper.plt.ylabel('time (sec)')
# save plot, beautify
plt_helper.savefig(fname)
assert os.path.isfile(fname + '.pdf'), 'ERROR: plotting did not create PDF file'
# assert os.path.isfile(fname + '.pgf'), 'ERROR: plotting did not create PGF file'
assert os.path.isfile(fname + '.png'), 'ERROR: plotting did not create PNG file'
return None
def main(cwd=''):
"""
Main driver
Args:
cwd (str): current working directory (need this for testing)
"""
# Loop over variants, exact and inexact solves
results = {}
for variant in ['fully-implicit', 'multi-implicit', 'semi-implicit']:
results[(variant, 'exact')] = run_SDC_variant(variant=variant, inexact=False)
results[(variant, 'inexact')] = run_SDC_variant(variant=variant, inexact=True)
# dump result
fname = cwd + 'data/timings_SDC_variants_Fisher'
file = open(fname + '.pkl', 'wb')
pickle.dump(results, file)
file.close()
assert os.path.isfile(fname + '.pkl'), 'ERROR: pickle did not create file'
# visualize
show_results(fname)
if __name__ == "__main__":
main()
Results:
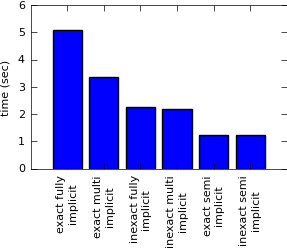
Full code: pySDC/projects/SDC_showdown/SDC_timing_GrayScott.py
import os
import pickle
import numpy as np
from petsc4py import PETSc
import pySDC.helpers.plot_helper as plt_helper
from pySDC.helpers.stats_helper import get_sorted
from pySDC.implementations.controller_classes.controller_nonMPI import controller_nonMPI
from pySDC.implementations.problem_classes.GrayScott_2D_PETSc_periodic import (
petsc_grayscott_multiimplicit,
petsc_grayscott_fullyimplicit,
petsc_grayscott_semiimplicit,
)
from pySDC.implementations.sweeper_classes.generic_implicit import generic_implicit
from pySDC.implementations.sweeper_classes.imex_1st_order import imex_1st_order
from pySDC.implementations.sweeper_classes.multi_implicit import multi_implicit
def setup_parameters():
"""
Helper routine to fill in all relevant parameters
Note that this file will be used for all versions of SDC, containing more than necessary for each individual run
Returns:
description (dict)
controller_params (dict)
"""
# initialize level parameters
level_params = dict()
level_params['restol'] = 1e-08
level_params['dt'] = 1.0
level_params['nsweeps'] = [1]
# initialize sweeper parameters
sweeper_params = dict()
sweeper_params['quad_type'] = 'RADAU-RIGHT'
sweeper_params['num_nodes'] = [3]
sweeper_params['Q1'] = ['LU']
sweeper_params['Q2'] = ['LU']
sweeper_params['QI'] = ['LU']
sweeper_params['initial_guess'] = 'zero'
# initialize problem parameters
problem_params = dict()
problem_params['Du'] = 1.0
problem_params['Dv'] = 0.01
problem_params['A'] = 0.09
problem_params['B'] = 0.086
problem_params['nvars'] = [(128, 128)]
problem_params['nlsol_tol'] = 1e-10
problem_params['nlsol_maxiter'] = 100
problem_params['lsol_tol'] = 1e-10
problem_params['lsol_maxiter'] = 100
# initialize step parameters
step_params = dict()
step_params['maxiter'] = 50
# initialize space transfer parameters
# space_transfer_params = dict()
# space_transfer_params['finter'] = True
# initialize controller parameters
controller_params = dict()
controller_params['logger_level'] = 30
# fill description dictionary for easy step instantiation
description = dict()
description['problem_class'] = None # pass problem class
description['problem_params'] = problem_params # pass problem parameters
description['sweeper_class'] = None # pass sweeper (see part B)
description['sweeper_params'] = sweeper_params # pass sweeper parameters
description['level_params'] = level_params # pass level parameters
description['step_params'] = step_params # pass step parameters
# description['space_transfer_class'] = mesh_to_mesh_petsc_dmda # pass spatial transfer class
# description['space_transfer_params'] = space_transfer_params # pass paramters for spatial transfer
return description, controller_params
def run_SDC_variant(variant=None, inexact=False, cwd=''):
"""
Routine to run particular SDC variant
Args:
variant (str): string describing the variant
inexact (bool): flag to use inexact nonlinear solve (or nor)
cwd (str): current working directory
Returns:
timing (float)
niter (float)
"""
# load (incomplete) default parameters
description, controller_params = setup_parameters()
# add stuff based on variant
if variant == 'fully-implicit':
description['problem_class'] = petsc_grayscott_fullyimplicit
description['sweeper_class'] = generic_implicit
elif variant == 'semi-implicit':
description['problem_class'] = petsc_grayscott_semiimplicit
description['sweeper_class'] = imex_1st_order
elif variant == 'multi-implicit':
description['problem_class'] = petsc_grayscott_multiimplicit
description['sweeper_class'] = multi_implicit
else:
raise NotImplementedError('Wrong variant specified, got %s' % variant)
if inexact:
description['problem_params']['lsol_maxiter'] = 2
description['problem_params']['nlsol_maxiter'] = 1
out = 'Working on inexact %s variant...' % variant
else:
out = 'Working on exact %s variant...' % variant
print(out)
# set time parameters
t0 = 0.0
Tend = 1.0
# instantiate controller
controller = controller_nonMPI(num_procs=1, controller_params=controller_params, description=description)
# get initial values on finest level
P = controller.MS[0].levels[0].prob
uinit = P.u_exact(t0)
# call main function to get things done...
uend, stats = controller.run(u0=uinit, t0=t0, Tend=Tend)
# load reference solution to compare with
fname = cwd + 'data/GS_reference.dat'
viewer = PETSc.Viewer().createBinary(fname, 'r')
uex = P.u_exact(t0)
uex[:] = PETSc.Vec().load(viewer)
err = abs(uex - uend)
# filter statistics by variant (number of iterations)
iter_counts = get_sorted(stats, type='niter', sortby='time')
# compute and print statistics
niters = np.array([item[1] for item in iter_counts])
out = ' Mean number of iterations: %4.2f' % np.mean(niters)
print(out)
out = ' Range of values for number of iterations: %2i ' % np.ptp(niters)
print(out)
out = ' Position of max/min number of iterations: %2i -- %2i' % (int(np.argmax(niters)), int(np.argmin(niters)))
print(out)
out = ' Std and var for number of iterations: %4.2f -- %4.2f' % (float(np.std(niters)), float(np.var(niters)))
print(out)
print('Iteration count (nonlinear/linear): %i / %i' % (P.snes_itercount, P.ksp_itercount))
print(
'Mean Iteration count per call: %4.2f / %4.2f'
% (P.snes_itercount / max(P.snes_ncalls, 1), P.ksp_itercount / max(P.ksp_ncalls, 1))
)
timing = get_sorted(stats, type='timing_run', sortby='time')
print('Time to solution: %6.4f sec.' % timing[0][1])
print('Error vs. reference solution: %6.4e' % err)
print()
assert err < 3e-06, 'ERROR: variant %s did not match error tolerance, got %s' % (variant, err)
assert np.mean(niters) <= 10, 'ERROR: number of iterations is too high, got %s' % np.mean(niters)
return timing[0][1], np.mean(niters)
def show_results(fname):
"""
Plotting routine
Args:
fname: file name to read in and name plots
"""
file = open(fname + '.pkl', 'rb')
results = pickle.load(file)
file.close()
plt_helper.mpl.style.use('classic')
plt_helper.setup_mpl()
plt_helper.newfig(textwidth=238.96, scale=1.0)
xcoords = list(range(len(results)))
sorted_data = sorted([(key, results[key][0]) for key in results], reverse=True, key=lambda tup: tup[1])
heights = [item[1] for item in sorted_data]
keys = [(item[0][1] + ' ' + item[0][0]).replace('-', '\n') for item in sorted_data]
plt_helper.plt.bar(xcoords, heights, align='center')
plt_helper.plt.xticks(xcoords, keys, rotation=90)
plt_helper.plt.ylabel('time (sec)')
# save plot, beautify
plt_helper.savefig(fname)
assert os.path.isfile(fname + '.pdf'), 'ERROR: plotting did not create PDF file'
# assert os.path.isfile(fname + '.pgf'), 'ERROR: plotting did not create PGF file'
assert os.path.isfile(fname + '.png'), 'ERROR: plotting did not create PNG file'
return None
def run_reference():
"""
Helper routine to create a reference solution using very high order SDC and small time-steps
"""
description, controller_params = setup_parameters()
description['problem_class'] = petsc_grayscott_semiimplicit
description['sweeper_class'] = imex_1st_order
description['sweeper_params']['num_nodes'] = 9
description['level_params']['dt'] = 0.01
# set time parameters
t0 = 0.0
Tend = 1.0
# instantiate controller
controller = controller_nonMPI(num_procs=1, controller_params=controller_params, description=description)
# get initial values on finest level
P = controller.MS[0].levels[0].prob
uinit = P.u_exact(t0)
# call main function to get things done...
uend, stats = controller.run(u0=uinit, t0=t0, Tend=Tend)
# filter statistics by variant (number of iterations)
iter_counts = get_sorted(stats, type='niter', sortby='time')
# compute and print statistics
niters = np.array([item[1] for item in iter_counts])
out = ' Mean number of iterations: %4.2f' % np.mean(niters)
print(out)
out = ' Range of values for number of iterations: %2i ' % np.ptp(niters)
print(out)
out = ' Position of max/min number of iterations: %2i -- %2i' % (int(np.argmax(niters)), int(np.argmin(niters)))
print(out)
out = ' Std and var for number of iterations: %4.2f -- %4.2f' % (float(np.std(niters)), float(np.var(niters)))
print(out)
print('Iteration count (nonlinear/linear): %i / %i' % (P.snes_itercount, P.ksp_itercount))
print(
'Mean Iteration count per call: %4.2f / %4.2f'
% (P.snes_itercount / max(P.snes_ncalls, 1), P.ksp_itercount / max(P.ksp_ncalls, 1))
)
timing = get_sorted(stats, type='timing_run', sortby='time')
print('Time to solution: %6.4f sec.' % timing[0][1])
fname = 'data/GS_reference.dat'
viewer = PETSc.Viewer().createBinary(fname, 'w')
viewer.view(uend)
assert os.path.isfile(fname), 'ERROR: PETSc did not create file'
return None
def main(cwd=''):
"""
Main driver
Args:
cwd (str): current working directory (need this for testing)
"""
# Loop over variants, exact and inexact solves
results = {}
for variant in ['fully-implicit', 'multi-implicit', 'semi-implicit']:
results[(variant, 'exact')] = run_SDC_variant(variant=variant, inexact=False, cwd=cwd)
results[(variant, 'inexact')] = run_SDC_variant(variant=variant, inexact=True, cwd=cwd)
# dump result
fname = 'data/timings_SDC_variants_GrayScott'
file = open(fname + '.pkl', 'wb')
pickle.dump(results, file)
file.close()
assert os.path.isfile(fname + '.pkl'), 'ERROR: pickle did not create file'
# visualize
show_results(fname)
if __name__ == "__main__":
# run_reference()
main()
Results:
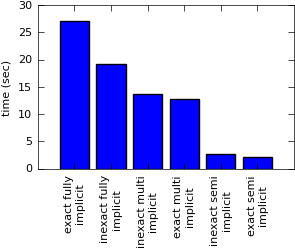